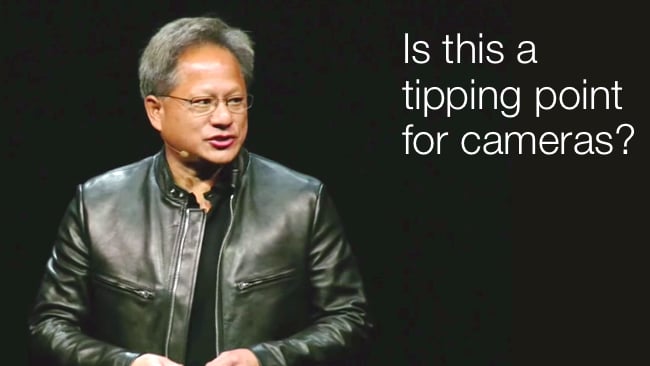
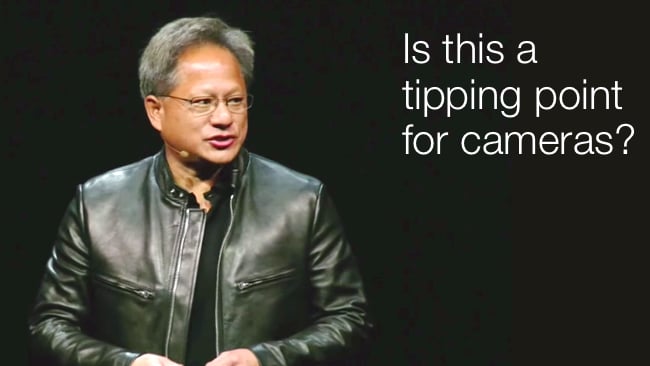
Hold on to your hats! It's starting to look like Artificial Intelligence is going to affect video production in a very big way.
Just a week or so, we awarded one of our RedShark NAB awards to iZotope, for their RX6 software, which uses Machine Learning to remove unwanted audio problems from dialogue tracks.
Fast forward to today, and I've just watched a presentation by Jensem Huang, President and CEO of Nvidia. This is a company that now makes a gigantic processing chip - again, designed with Machine Learning in mind - with TWENTY ONE BILLION transistors in it. On a single chip, that's extraordinary and right at the limits of what's possible. Apparently the yield (the percentage of chips that work from a single slice of silicon) is very low.
Apart from this humungous processor, we learned from the keynote presentation that Nvidia is employing Machine Learning in the quest to speed up Ray Tracing. Stay with me here - because if you understand this piece of the jigsaw, then you'll see why it's important for cameras of the future.
Ray Tracing is a laborious process that plots the path of every single light beam in a computer-generated scene. It's slow, but absolutely the best way to create photorealistic objects and environments. How slow? As slow as you have the patience to type the word "very" multiple times. It really needs speeding up if we're going to see it used outside of facilities that have extremely powerful render farms.
One problem that emerges from this slowness is that for designers rendering with ray tracing, it takes ages even to visualise their creations. So most renderers will allow you to preview the image at extremely reduced resolutions. This speeds things up a lot, but at the cost of quality. Even though the images improve the longer you leave them, it makes for a tedious workflow.
Now, it seems, with the help of machine learning, it's possible for a computer or processor to "guess" at what the final image will be like. It bases these guesses on hundreds (or millions) of scenes that is shown during training.
So the "machine" learns, sort of, what things look like. If it sees a circle-like thing, it can draw an accurate circle. If it sees a steering wheel, it will draw a steering wheel, even if there isn't enough information to do it conventionaly. Noise won't matter any more than it matters when we recognise our own house when it's snowing. We're not shown what it will do with human faces - and that will be a big test.
Essentially what's going on here is making sense out of disorder. It's noise reduction by assuming what's there through the randomness. It's an assumption based on - we might almost say "knowledge". It's based on stuff that the "machine" has learned.
Seeing this (it's at 1:45 into the video) makes me wonder if this is a pivotal time for video.
Is this the moment we should start asking for cameras that don't just see the world, but understand it as well? If it is, then we will be able to move beyond, 4K, 8K and even 16K (which I don't think will ever happen) and record video that doesn't use pixels at all.
(I'm not completely sure who to thank for this video clip. It's branded "Engadget" - so thanks to them for bringing it to my attention.)
Tags: Technology
Comments